Future-Proofing SMB & Commercial Lending: Leveraging Financial Forecasting and Scenario Analysis in Credit Underwriting
- Dony
- Apr 11
- 8 min read
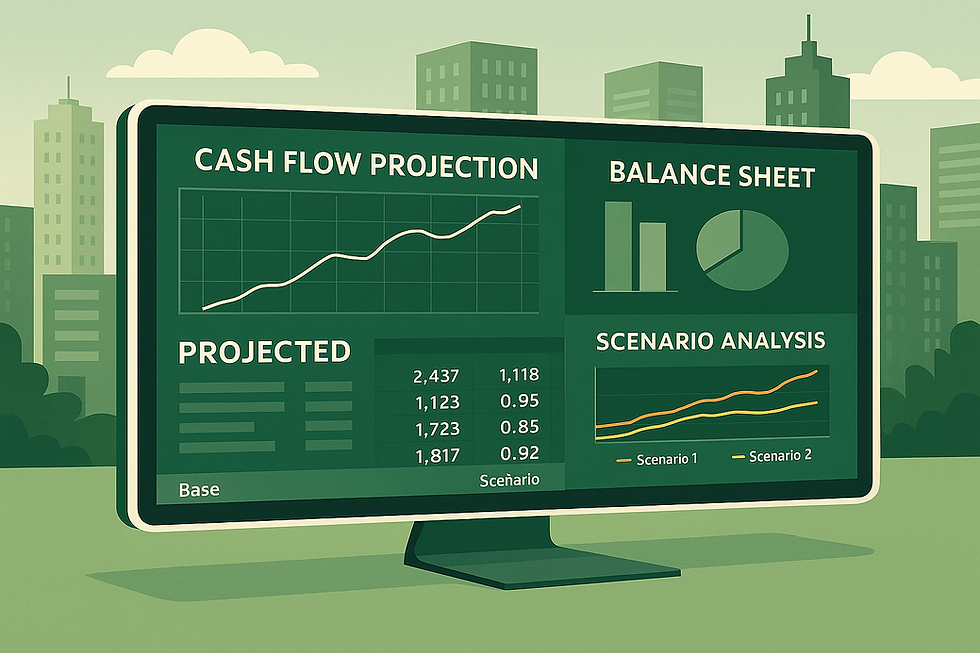
The Imperative for Forward-Looking Credit Assessment
The Lending process for small-to-medium businesses (SMBs) and corporate is obsolete and has not changed for the past decade; The process is still based on historical financial information. Economic volatility, shifting market dynamics, and unforeseen global events mean that lenders relying solely on historical data face significant challenges. Traditional credit risk assessments, often based on static, backward-looking information, can prove insufficient in gauging a borrower's true capacity to navigate future uncertainties and meet their obligations.
This evolving environment necessitates a more proactive and forward-looking approach to credit underwriting. Financial forecasting and scenario analysis represent critical tools to achieve that. By projecting potential future financial performance and assessing business resilience under various conditions, these methodologies allow lenders to move beyond simple historical analysis. They enable a more nuanced understanding of risk, support more informed decision-making, and ultimately help build more resilient lending portfolios.
This article explores how integrating financial forecasting and scenario analysis can significantly enhance the credit underwriting process for SMB and corporates in commercial lending.
Understanding Financial Forecasting: Projecting Future Financial Health
In the context of credit underwriting, financial forecasting involves predicting a borrower's likely future financial performance and position. It translates assumptions about future business activity, market conditions, and management decisions into quantitative projections.
Type of Forecasting: Dynamic vs. Static:
Static Forecasting: Creates a projection for a fixed future period (e.g., the next financial year) based on assumptions made at a single point in time. It does not automatically update as new information becomes available within that period.
Dynamic Forecasting (or Rolling Forecast): Is continuously updated (e.g., monthly or quarterly) as actual results come in and assumptions are revised. It typically maintains a consistent forecast horizon (e.g., always looking 12 months ahead).
Advantages of Dynamic: Dynamic forecasting offers greater agility and responsiveness. It allows lenders to react more quickly to changing circumstances, providing a more current view of expected performance and potential risks.
Relevance of Static: Static forecasts may still be suitable for certain situations, such as specific project finance assessments with clearly defined timelines or for businesses operating in highly stable, predictable environments where assumptions are unlikely to change significantly over the forecast period.
Financial Statements Forecasted: Effective forecasting typically involves projecting the core financial statements:
Income Statement: Predicts future revenues, costs, and ultimately, profitability (Net Income).
Balance Sheet: Projects future assets, liabilities, and equity, showing the expected financial position.
Cash Flow Statement: Forecasts cash inflows and outflows from operating, investing, and financing activities, crucial for assessing liquidity and debt servicing capacity.
Main Lines Forecasted: Within these statements, key line items are projected, including:
Income Statement: Revenue/Turnover, Cost of Goods Sold (COGS), Gross Profit, Operating Expenses (SG&A), EBITDA, Interest, Taxes, and Net Income.
Balance Sheet: Assets (Cash, Accounts Receivable, Inventory, Fixed Assets), Liabilities (Accounts Payable, Short-term Debt, Long-term Debt), and Equity.
Cash Flow Statement: Cash from Operations, Capital Expenditures, Debt Repayments, Equity Issuances/Repurchases, and Net Change in Cash.
Horizon of Forecasts: The timeframe for forecasts varies:
Short-term (0-12 months): Focuses on immediate liquidity, working capital needs, and operational performance.
Medium-term (1-3 years): Often aligns with typical loan terms, assessing profitability trends and debt repayment capacity over that period.
Long-term (3+ years): Considers strategic viability, long-term debt structures, and sustainable growth potential. The appropriate horizon depends on factors like the loan's purpose and tenor, the borrower's industry and business cycle, and the reliability of long-range assumptions.
Scenario Analysis: Exploring Potential Future Pathways
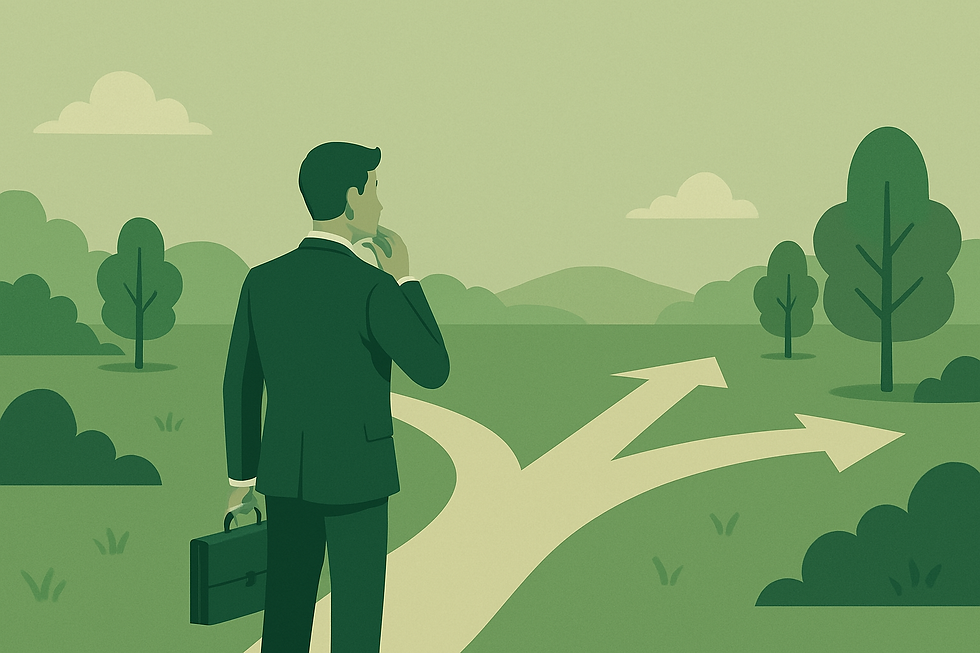
While forecasting aims to predict the most probable financial outcome, scenario analysis explores the potential impact of different future events or conditions on a borrower's financial health. It is a method for assessing risk and resilience by considering multiple possible futures.
Type of Scenario Analysis:
Deterministic vs. Stochastic: Deterministic analysis usually involves assessing a few specific, clearly defined scenarios (e.g., base, best, worst case) with single-point outcome estimates. Stochastic analysis, often using techniques like Monte Carlo simulations, assigns probabilities to various inputs to generate a distribution of potential outcomes, providing a richer view of risk.
Stress Testing: A specific type of scenario analysis focused on evaluating a borrower's resilience under severe, adverse conditions (e.g., deep recession, sharp interest rate hikes, major customer loss).
Scenario Categories: Scenarios can be grouped based on their drivers:
Case-based: Best-case, Worst-case, Base-case (often linked to the primary forecast).
Driver-based: Macroeconomic (GDP growth, inflation, interest rates), Industry-specific (regulatory changes, technological shifts, competitive pressures), Company-specific (management changes, operational issues, key contract wins/losses).
Difference and Similarity between Financial Forecasting and Scenario Analysis:
Key Difference: Financial forecasting typically produces a single set of projections representing the most likely or expected outcome based on a defined set of assumptions. Scenario analysis, conversely, does not predict the future but explores multiple possible futures by altering key assumptions from the base forecast. Forecasting answers "What is likely to happen?", while scenario analysis answers "What might happen if...?"
Similarity: Both are inherently forward-looking techniques that rely on analysing financial data and making assumptions about the future to inform decision-making. Scenario analysis often uses the financial forecast as its starting point or baseline.
The Synergy: How Financial Forecasting and Scenario Analysis Interconnect
Financial forecasting and scenario analysis are not isolated techniques; they work most effectively in tandem.
Baseline Provision: The financial forecast provides the essential baseline projection – the expected financial path under a given set of core assumptions.
Exploring Deviations: Scenario analysis takes this baseline forecast and applies different sets of assumptions (representing various potential positive or negative events) to the model. This allows lenders to see how the projected Income Statement, Balance Sheet, and Cash Flow Statement might change under different circumstances, revealing potential vulnerabilities or opportunities.
Comprehensive Risk Assessment: Forecasting offers the central estimate ("most likely" path), while scenario analysis maps out the potential variability around that path ("what ifs"). Together, they provide a more comprehensive understanding of the potential range of outcomes and the associated risks, moving beyond a single point estimate.
Dynamic Modelling: This synergy is often embodied in dynamic financial models where a base-case forecast is established, alongside pre-defined upside (best-case) and downside (worst-case or stress) scenarios. These allow for quick assessment of potential impacts as conditions evolve.
Sensitivity Analysis: A related technique, sensitivity analysis, complements this by isolating the impact of changing a single key assumption (e.g., sales growth rate, key input cost) on the financial forecast, helping to identify the most critical drivers of performance and risk.
Technical Foundations: From Traditional Statistics to AI
Generating reliable financial forecasts requires robust methodologies and careful handling of data, leveraging both established statistical techniques and cutting-edge artificial intelligence.
Traditional Forecasting Methods: Established statistical approaches remain valuable foundations for forecasting:
Time Series Analysis: Techniques like ARIMA (AutoRegressive Integrated Moving Average) and Exponential Smoothing analyse historical patterns (trends, seasonality) within a data series (e.g., past sales) to project future values.
Regression Analysis: Establishes relationships between financial variables (e.g., revenue) and other internal or external factors (e.g., marketing spend, GDP growth, industry indicators) to build predictive models.
Core Technical Aspects: Regardless of the specific method, certain technical aspects are crucial:
Data History and Quality: Accurate forecasting relies heavily on sufficient (typically several years) and reliable historical financial data. The quality and consistency of this data are paramount.
Seasonality: Identifying and incorporating recurring seasonal patterns (e.g., higher sales in Q4 for retailers) into the forecast model is essential for accuracy.
Training, Testing, Validating: Forecasting models must be rigorously validated. This typically involves training the model on one part of the data, testing its predictions on unseen data, and validating its historical accuracy (e.g., backtesting). Measuring forecast error helps quantify reliability.
Data Gaps and Outliers: Strategies are needed to handle missing data points and identify/address outliers that could distort future projections.
Advanced AI-Driven Forecasting: While traditional methods are effective, artificial intelligence (AI) and machine learning (ML) are bringing new levels of sophistication and predictive power:
Recent AI Techniques: AI/ML algorithms excel at identifying complex, non-linear patterns. Techniques like neural networks, deep learning (e.g., LSTMs, GRUs), gradient boosting machines, and even Transformer models are increasingly applied for enhanced accuracy.
State-of-the-Art Tools: Prominent libraries and frameworks facilitate advanced forecasting, including GluonTS, Prophet, AutoTS, Darts, and Temporal Fusion Transformers (TFT). These tools often incorporate probabilistic forecasting (outputting a range of outcomes and their likelihoods) rather than just single point estimates.
Enhanced Capabilities: AI/ML enables automatic feature engineering, ensemble methods, and better handling of large, complex datasets. Techniques involving synthetic data generation can help in limited data scenarios, while AutoML platforms increase accessibility. Cloud providers offer sophisticated, managed AI/ML forecasting services.
Reflecting Scenarios on Financial Statements: Impact and Examples
Scenario analysis translates hypothetical events or assumption changes into tangible impacts on the borrower's projected financials.
Linking Scenarios to Financial Statements: The process involves adjusting specific line items within the baseline financial forecast based on the scenario's assumptions. For instance:
A recession scenario might assume a -10% change in projected Revenue. This decrease would flow through to Gross Profit and, after adjusting relevant variable costs and potentially increasing bad debt provisions (an expense), impact Operating Profit and Net Income.
On the Balance Sheet, lower sales might lead to lower Accounts Receivable (but perhaps a higher proportion of doubtful accounts), potentially slower inventory turnover, and implications for cash levels.
The Cash Flow Statement would reflect the reduced cash inflow from operations due to lower profits and potentially slower customer payments.
Examples:
Recession Scenario: Assume a significant drop in GDP growth. This translates to lower forecasted Revenue, potentially higher Cost of Goods Sold if input costs become sticky or suppliers face distress, increased provisions for bad debts impacting Operating Expenses, and consequently, lower Net Income and reduced operating cash flow. Debt covenants (e.g., Debt Service Coverage Ratio) might come under pressure.
Interest Rate Hikes: Assume central bank rates increase sharply. This directly increases the Interest Expense line on the Income Statement for variable-rate debt, reducing Net Income. It also increases cash outflows for debt servicing on the Cash Flow Statement, potentially straining liquidity.
Supply Chain Disruption: Assume a key supplier fails or shipping costs surge. This could lead to reduced Revenue (if goods cannot be produced/delivered), increased Cost of Goods Sold, higher Inventory levels (if finished goods cannot ship or raw materials are stockpiled), impacting profitability and working capital reflected across all three statements.
Case Studies: Lenders might model the impact of a 2% increase in base rates on their entire commercial loan portfolio's projected interest coverage ratios, identifying borrowers most at risk. Or, they might simulate the effect of a sector-specific downturn (e.g., travel industry during a pandemic) on relevant borrowers' projected revenues and cash flows to anticipate potential defaults.
Integration and Business Impact: Enhancing Workflow and Outcomes

Effectively leveraging financial forecasting and scenario analysis requires seamless integration into the credit underwriting workflow, yielding significant business benefits beyond simple risk mitigation.
Integrating into the Credit Lifecycle: These forward-looking tools add value at multiple stages:
Pre-Approval: Assess initial viability and repayment capacity under various conditions.
Setting Terms: Inform decisions on loan amount, pricing, and covenants based on projected performance and stress tests.
Credit Limits: Dynamically adjust exposure, especially for revolving facilities, based on updated forecasts.
Ongoing Monitoring & Portfolio Management: Regularly update forecasts for existing borrowers to proactively identify emerging risks or distress signals, allowing for early intervention well before issues escalate. Use forward-looking insights for periodic credit reviews.
Balancing Automation and Judgement: While technology drives the modelling, human oversight remains critical. Experienced analysts must set realistic assumptions, interpret outputs in context, and apply nuanced judgement. The goal is augmented intelligence, enhancing, not replacing, analyst expertise.
Tangible Business Benefits: Integrating these techniques drives improved outcomes:
More Accurate Decisions & Reduced Losses: Better risk assessment leads to fewer defaults and lower loan losses, improving portfolio quality and Net profitability.
Identifying Opportunities: Deeper insight into borrower resilience can uncover sound lending opportunities that might otherwise be missed.
Optimised Pricing: Granular risk understanding under different scenarios enables more sophisticated risk-based pricing.
Stronger Borrower Relationships: Proactive engagement based on forward-looking analysis fosters trust, collaboration, and positions the lender as a strategic partner.
Conclusion: Building the Future of Credit Assessment with Crediflow.AI
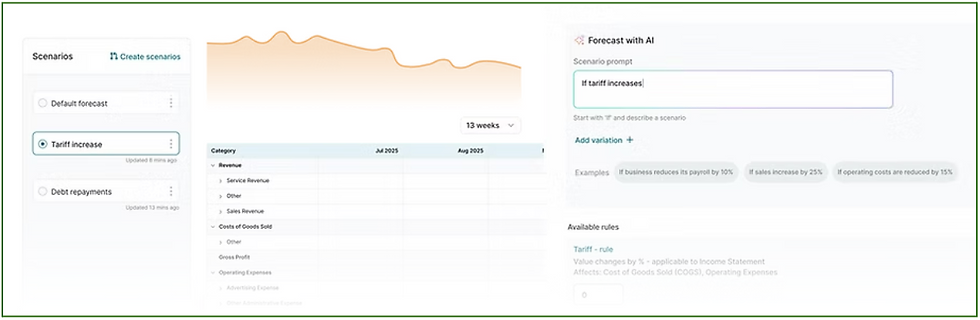
Modern SMB and commercial lending requires a fundamental change towards proactive, predictive insights. As we've explored, financial forecasting and scenario analysis are essential tools in achieving this, offering deeper understanding of borrowers' future financial performance and enabling more confident decision-making.
At Crediflow.AI, we recognise that implementing these capabilities can seem complex amidst outdated processes and manual reviews. Our mission is to transform business lending, making sophisticated credit assessment seamless and efficient. We have integrated robust financial forecasting and dynamic scenario analysis directly into our platform, moving beyond static data points to provide a real-time, 360-degree view of business financial health which enables faster, fairer, and more accurate credit assessments.
Discover how Crediflow.AI can help transform your lending operations. Visit crediflow.ai to learn more.
Comentários